
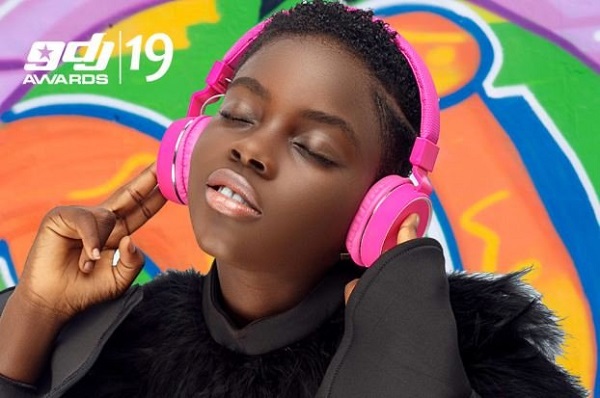
In the context of maMIMO power allocation, adversarial attacks refer to the injection of subtle perturbations into the deep learning model’s input, during inference (i.e., the adversarial perturbation is injected into inputs during deployment after the model has been trained) that are specifically crafted to force the trained regression model to output an infeasible power allocation solution. Yet, such deep learning models are vulnerable to adversarial attacks.
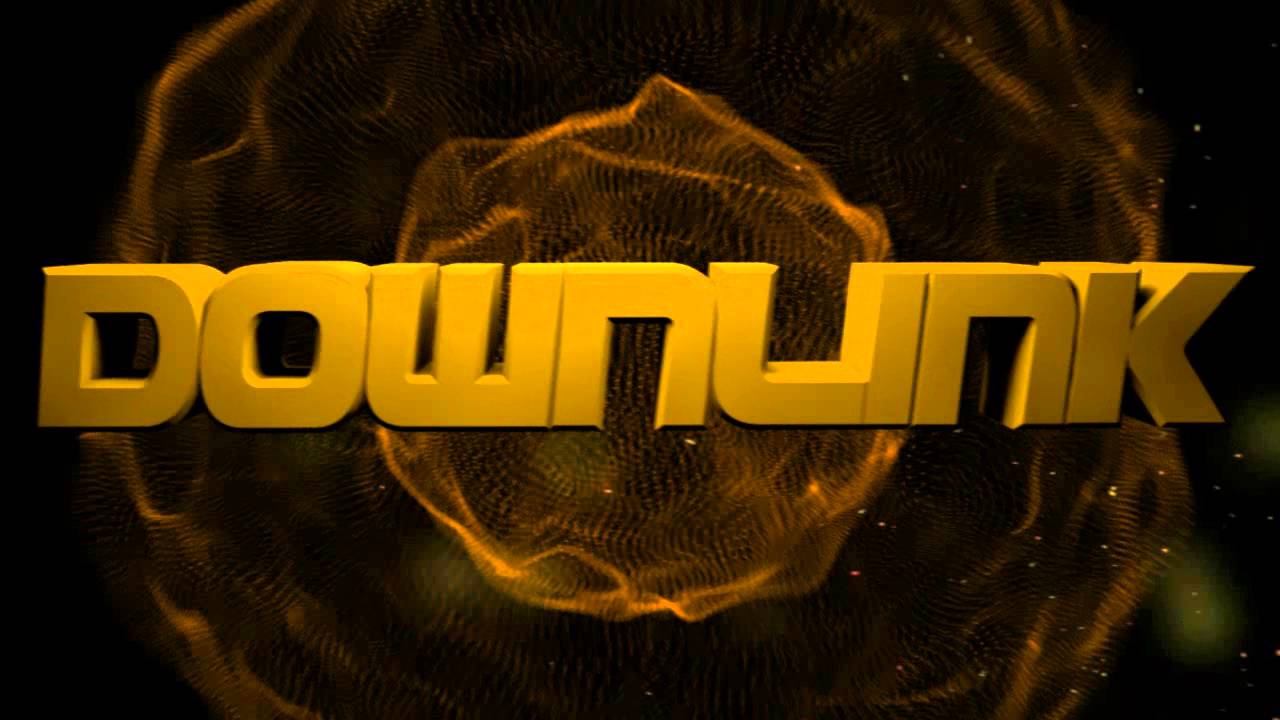
N2 - Recent work has advocated for the use of deep learning to perform power allocation in the downlink of massive MIMO (maMIMO) networks. T1 - Defending Adversarial Attacks on Deep Learning-Based Power Allocation in Massive MIMO Using Denoising Autoencoders We test our defense across multiple attacks and in multiple threat models and demonstrate its ability to (i) mitigate the effects of adversarial attacks on power allocation networks using two common precoding schemes, (ii) outperform previously proposed benchmarks for mitigating regression-based adversarial attacks on maMIMO networks, (iii) retain accurate performance in the absence of an attack, and (iv) operate with low computational overhead.", Specifically, we develop a denoising autoencoder (DAE), which learns a mapping between potentially perturbed data and its corresponding unperturbed input.
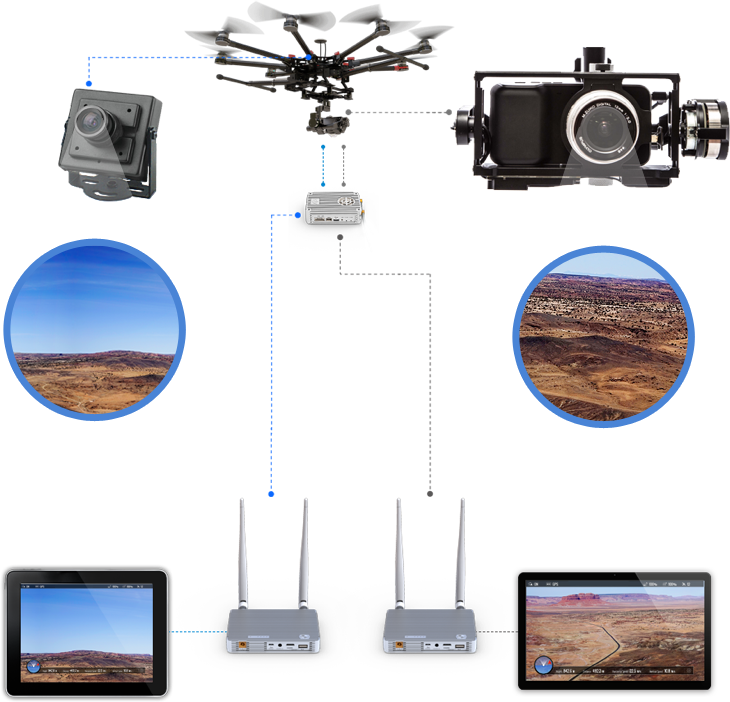
In this work, we develop an autoencoder-based mitigation technique, which allows deep learning-based power allocation models to operate in the presence of adversaries without requiring retraining. In the context of maMIMO power allocation, adversarial attacks refer to the injection of subtle perturbations into the deep learning models input, during inference (i.e., the adversarial perturbation is injected into inputs during deployment after the model has been trained) that are specifically crafted to force the trained regression model to output an infeasible power allocation solution. We test our defense across multiple attacks and in multiple threat models and demonstrate its ability to (i) mitigate the effects of adversarial attacks on power allocation networks using two common precoding schemes, (ii) outperform previously proposed benchmarks for mitigating regression-based adversarial attacks on maMIMO networks, (iii) retain accurate performance in the absence of an attack, and (iv) operate with low computational overhead.Ībstract = "Recent work has advocated for the use of deep learning to perform power allocation in the downlink of massive MIMO (maMIMO) networks. Recent work has advocated for the use of deep learning to perform power allocation in the downlink of massive MIMO (maMIMO) networks.
